Explore the future of data and application observability: AI-powered monitoring, end-to-end visibility, and critical trends shaping how to ensure system reliability.
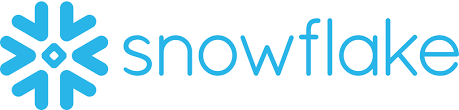
The data and application observability landscape has dramatically transformed in recent years. As enterprises increasingly rely on complex, distributed systems and AI-driven solutions, the need for comprehensive monitoring and troubleshooting tools has never been greater. This evolution has given rise to a new generation of startups and technologies dedicated to meeting the growing observability needs of enterprises in an AI-driven world.
Snowflake has strategically invested in emerging application and data observability solutions providers to build applications on the AI data cloud.
The Rise of Data and Application Observability
The explosion of data and the proliferation of microservices architecture have made traditional monitoring approaches insufficient. Observability has become critical, enabling developers and engineers to gain deep insights into their systems' behavior and performance. This shift has led to significant innovations in monitoring and troubleshooting in modern data environments.
Key Trends Shaping the Future of Observability
1. AI-Powered Monitoring and Alerting
One of the most significant trends in observability is integrating artificial intelligence to enhance monitoring capabilities. Jeremy Burton, CEO of Observe, highlights this trend: "We're very bullish on the potential of GenAI to make observability more accessible to more, less technical, users. Our new AI Investigator orchestrates a network of domain-specific AI agents to assist on-call engineers in quickly identifying and resolving issues."
This AI-driven approach is not unique to Observe. Amy Reams, VP of Business Development and Marketing at Anomalo, explains how their platform leverages AI for data quality monitoring: "Anomalo uses unsupervised machine learning models to monitor deeper data quality issues caused by changes in the data values themselves. This unique capability enables data quality at scale, reducing the time teams spend writing manual rules or tests."
2. End-to-End Observability
As data pipelines become more complex, there's a growing need for observability solutions to provide visibility across the entire data lifecycle. Kevin Hu, Co-founder and CEO of Metaplane, emphasizes this point: "Metaplane integrates across the product ecosystem to monitor not just the data within the data warehouse but also the processes affecting it—whether it's ingestion through data pipelines, transformations, or consumption in notebooks. This provides an end-to-end observability experience that covers data ingestion, transformation, and consumption."
This holistic approach to observability is becoming increasingly important as organizations seek to understand and manage their entire data stack.
3. Native Integration and Ease of Use
A key trend in the observability space is the move towards more integrated, easy-to-use solutions. Lior Gavish, co-founder and CTO of Monte Carlo, notes: "We were intentionally drawing a parallel to software engineering and application performance management. At the time, software engineering had much more mature processes and solutions for ensuring the reliability of their IT applications than data engineers had for their data applications."
This push for more integrated solutions drives innovations in deploying and managing observability tools. Many providers now offer native applications or one-click installations that significantly reduce the complexity of setting up comprehensive observability systems.
4. Focus on Data Quality and Reliability
As organizations become more data-driven, ensuring the quality and reliability of data has become paramount. Amy Reams from Anomalo emphasizes this: "Modern data-driven organizations are using platforms to centralize all their data, making it readily available for everything from business decision-making to generative AI applications. But this also introduces more complexities as it increases the volume, velocity, and variety of data,, which leads to a growing number of data quality issues."
This focus on data quality drives the development of more sophisticated monitoring and alerting systems that automatically detect anomalies and potential issues in data pipelines.
5. Scalability and Performance
As data volumes grow exponentially, observability solutions must evolve to handle this increased scale without compromising performance. Kevin Hu from Metaplane notes: "Anything we can take advantage of in their platform means that we don't have to build it ourselves—over the long term, this will become a key competitive advantage."
This focus on scalability and performance drives innovations in collecting, storing, and analyzing observability data. Many providers are leveraging cloud-native architectures to achieve the necessary scale.
6. Security and Compliance
With the increasing focus on data privacy and regulatory compliance, observability solutions are evolving to address these concerns. Jeremy Burton from Observe states: "We're focusing on emerging standards like OpenTelemetry and technologies like Apache Iceberg. This work is critical. Over the past decade, customers have been locked due to proprietary agents collecting and storing data in proprietary databases. All this is about to change."
This shift towards open standards and improved data governance is helping organizations maintain compliance while gaining the benefits of comprehensive observability.
Implications for Developers, Engineers, and Architects
For professionals working with data and applications, these trends in observability have several important implications:
Embrace AI-powered tools: Look for observability solutions that leverage AI to provide more intelligent insights and automate problem detection.
Prioritize end-to-end visibility: Seek out tools that provide comprehensive visibility across your entire data and application stack.
Consider ease of integration: Evaluate observability solutions based on how easily they can be integrated into your existing infrastructure and workflows.
Focus on data quality: Implement robust data quality monitoring foundational to your observability strategy.
Scale plan: Ensure your chosen observability solutions can scale with your data and application growth.
Address security and compliance: Your observability tooling should include security features and compliance capabilities.
Conclusion: Navigating the Future of Observability
The convergence of advanced AI capabilities, cloud-native architectures, and the growing complexity of modern data systems is shaping the future of observability. As these trends evolve, we expect to see even more innovations in monitoring, troubleshooting, and optimizing our data and applications.
By staying informed about these trends and leveraging the advanced capabilities offered by modern observability platforms, organizations can ensure they're well-equipped to handle the observability challenges of tomorrow's data-driven world. The key lies in adopting powerful, comprehensive, and flexible solutions to adapt to the rapidly changing technological landscape.
Comments